Accurrate Asset Locating in Indoor Spaces with RF Fingerprinting
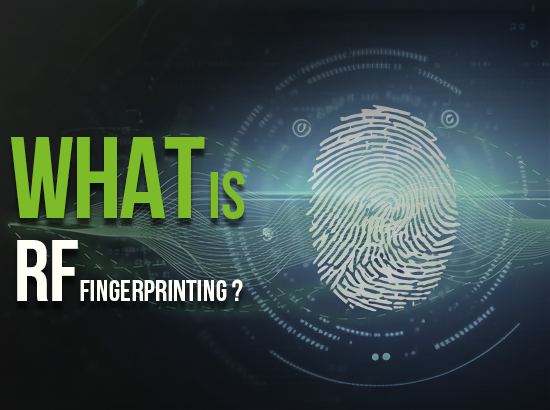
Indoor Locating Systems (ILS) that use radio signals have become
increasingly popular in recent years due to their revolutionary potential in a
variety of industries and applications. The preference for UWB (Ultra-Wideband) technology stems from its high bandwidth,
frequency density, and ability to provide centimeter-level accuracy in complex
indoor environments with obstacles.
Achieving high positioning accuracy in indoor spaces with complex geometries, multiple buildings, irregular shapes, and obstacles, such as manufacturing facilities and warehouses, necessitates advanced technological equipment and field experience. To achieve this precision, a large number of anchors and adequate Line-of-Sight (LOS) field coverage are required, which can present challenges in terms of effective cost management.
What is an RF fingerprint, and how is it used?
An RF(Radio Frequency) fingerprint identifies a device or communication signal based on its unique characteristics. RF fingerprinting identifies a device by analyzing the characteristics of the electromagnetic signals it emits or detects.
The unique identification parameters for an RF fingerprint are based on a set of features associated with the device's RF signals. These features, by associating them with the characteristics of the electromagnetic signals emitted or detected by the device, enable more precise tracking of entity locations and achieve better results in task and maintenance optimizations than other protocols.
How is RF fingerprint technology used for asset tracking?
RF fingerprint-supported asset localization technology achieves high-precision positioning in partially Line-of-Sight (LOS) indoor scenarios by combining trilateration and device fingerprint identities. During the offline phase, auxiliary anchors are used to create a dense fingerprint database, which is then used in the online phase to estimate distances between the tracked asset and the auxiliary anchors. This method can achieve centimeter-level accuracy in studies using UWB-enabled devices, saving money by reducing the number of deployed anchors.
What are the unique parameters for RF fingerprinting of a device?
A device generates unique identification parameters for RF fingerprints by combining variables such as frequency, power level, frequency shift, modulation type, signal attenuation, and so on. Devices use RF fingerprints for authentication, security, and precise location tracking.
• Frequency: In RF fingerprinting, frequency is the number of RF signals emitted or detected by a device. The operating frequency of each device can be custom-set. This frequency data is used to estimate the exact location of the device.
• Power Level: This refers to the transmission power of a device's RF signals. Each device's transmission power can vary or be parametrically defined. This parameter is considered an indicator for determining the device's location. Devices in close proximity typically have higher power levels
• Frequency Shift: Frequency shift refers to the deviation of RF signals from the desired frequency. Devices can have different frequency shifts, and this parameter is used to determine their unique identities.
• Modulation Type: The modulation type determines how RF signals are
carried and the transmission format. Each device may use a different modulation
type. This is an important factor in distinguishing devices from one another.
• Signal Attenuation: Signal attenuation refers to the power loss
that RF signals experience during transmission. Each device's signal
attenuation may vary. This parameter is considered when creating unique RF
fingerprints for devices.
• Waveform: The wave shape of radio
frequency (RF) signals. Different devices with different waveform types can
provide distinct identification. Various waveform shapes, such as sinusoidal,
square, and triangular waves, can be used.
• Delay Time: Delay time refers to the propagation or transmission
delay of RF signals. Each device may have a different delay time. This
parameter distinguishes devices from one another.
Improving efficiency and accuracy through RF fingerprinting & machine learning
The combination of machine learning and RF fingerprint technology allows for the development of smarter, more precise, and efficient business processes in production and warehouses. This provides significant advantages in industrial activities.
ü Asset Identification: By analyzing RF
signatures, machine learning can automatically recognize and classify assets.
This allows for fast and precise asset identification processes in production
lines and warehouses.
ü Positioning and tracking: By combining RF fingerprint technology with machine learning, assets can be more accurately positioned and tracked. The analysis and learning process for changes in RF signatures enables more precise tracking of asset location and movement. This greatly improves inventory management, logistics optimisation, and process efficiency.
ü Anomaly detection and prediction: Machine learning algorithms can detect anomalies and predict faults using RF fingerprint data. This allows for the early detection of malfunctions in manufacturing processes and the development of preventive maintenance strategies.
ü Real-time data analysis: Machine learning can process RF fingerprint data in real time. Algorithm-based analysis of constantly changing data can yield meaningful results. This facilitates rapid decision-making and immediate intervention.
ü Predictive analytics and productivity optimisation:
The combination of machine learning and RF fingerprinting allows for predictive
analytics. Future trends, demand, and the most efficient use of resources can
all be forecasted using historical data and RF signatures. This leads to more
effective planning, inventory management, and process efficiency.
The Key to Industrial Innovation: Combining RF Fingerprinting, Machine Learning, & UWB!
As a result, advanced technologies such as RF fingerprinting, machine learning, and UWB are enabling a significant shift in the industry. This combination combines UWB's high accuracy and positioning capabilities with RF fingerprinting technology developed to analyze and track devices' unique identification parameters. Machine learning algorithms improve learning and prediction capabilities by processing collected data. The combination of these three technologies makes industrial businesses smarter, more efficient, and more secure. It provides significant benefits in a variety of areas, including object and asset tracking, inventory management, manufacturing process optimization, and security protocol strengthening. The combination of RF fingerprinting, machine learning, and UWB technology aims to improve productivity and efficiency in business.
Industrial enterprises can improve operational
efficiency and resource management by leveraging Wipelot's
technologies in product, stock, and semi-finished product tracking, resource
management, and occupational safety. Real-time data is available thanks to
UWB-based geolocation and precise tracking, allowing businesses to optimise
inventory control, production tracking, and operational processes.
Contact us to learn more about
Wipelot's digital transformation solutions for your business. Meet the
innovative technologies that will shape your company's future.